Cryptocurrency price prediction incorporates advanced machine learning techniques, with LSTM networks consistently outperforming traditional statistical methods. Models integrating sentiment analysis from social media platforms, particularly Twitter/X, achieve accuracy rates up to 98.75% for major cryptocurrencies. External data sources, including Google Trends patterns and tweet volumes, greatly enhance forecasting reliability through multi-dimensional analysis. Technical indicators like RSI, EMA, and SMA, when combined with AI frameworks, offer investors increasingly refined predictive capabilities across varying market conditions and time horizons.
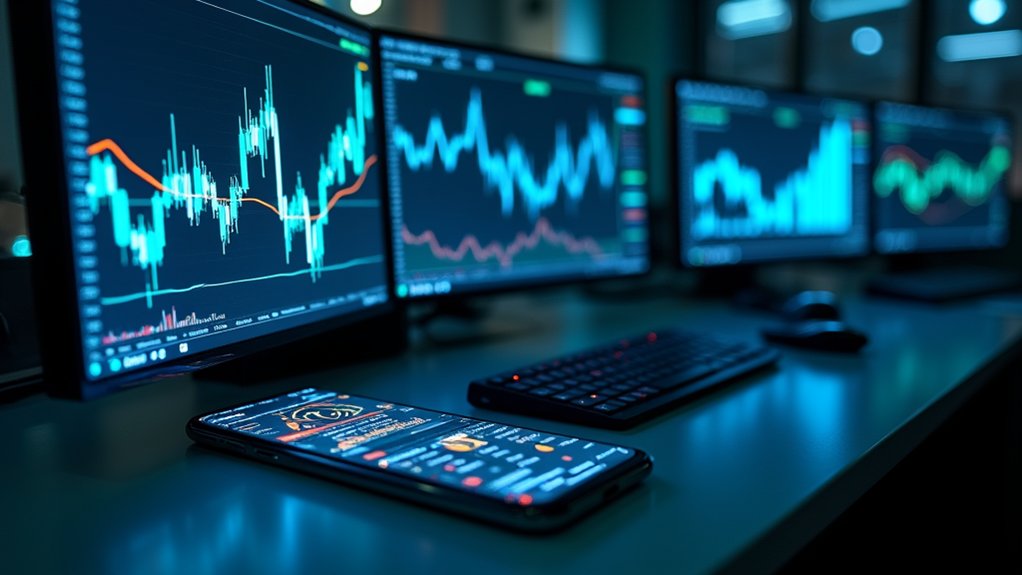
While traditional financial markets have long relied on statistical models for forecasting, the cryptocurrency sector has emerged like a fertile testing ground for advanced predictive technologies, particularly those leveraging artificial intelligence and machine learning frameworks.
Recent research demonstrates remarkable achievements in this domain, with CNN-based cryptocurrency prediction models attaining 98.75% accuracy when forecasting Bitcoin, Ethereum, Litecoin, and Monero prices across varying time horizons. These models consistently outperform conventional methodologies, exhibiting noticeably lower error metrics including RMSE, MAE, and MSE, while successfully integrating visualization tools for performance benchmarking.
The volatility characteristic of cryptocurrency markets appears inextricably linked to sentiment expressed across social media platforms, particularly Twitter/X, where public opinion frequently triggers price movements. The optimization of deep learning algorithms combined with sentiment analysis provides a comprehensive framework for capturing market dynamics influenced by social media trends. Researchers have responded by developing hybrid approaches that combine sentiment analysis tools like VADER and TextBlob with market data, though the reliability of these sentiment indicators tends to fluctuate during market downturns when objective tones predominate in online discourse.
Among deep learning architectures, LSTM networks have demonstrated superior performance when compared to alternative models including RNN, GRU, and CONV1D structures, achieving RMSE values like 4.21 on Bitcoin datasets. Bi-directional LSTMs and ensemble methods show particular promise for multi-hour predictions by capturing non-linear temporal correlations that often elude traditional machine learning approaches. The application of time series data analysis is crucial for recognizing patterns that indicate potential growth in specific cryptocurrencies.
External data sources greatly enhance predictive models, with Google Trends data and tweet volumes exhibiting strong correlations with both cryptocurrency adoption rates and price movements. Technical discussions regarding blockchain technology on social media indirectly influence market sentiment, while volumetric metrics frequently outperform pure sentiment analysis during price declines.
For investors and researchers seeking ideal prediction frameworks, Random Forest, Gradient Boosting, and LSTM models represent the most promising candidates for long-term forecasting, especially when enhanced with technical indicators like RSI, EMA, and SMA.
These indicators, when subjected to permutation importance analysis, reveal cryptocurrency-specific patterns that further refine predictive accuracy across diverse market conditions and time frames.
Frequently Asked Questions
Which Technical Indicators Are Most Reliable for Cryptocurrency Predictions?
Research indicates that RSI and MACD remain the most reliable technical indicators for cryptocurrency predictions, particularly when combined to mitigate false signals.
LSTM models incorporating these indicators have achieved over 86% accuracy in Bitcoin forecasting scenarios.
Additionally, moving averages, especially EMAs which respond more rapidly to market shifts, demonstrate strong predictive support when analyzed within appropriate timeframes and with consideration for cryptocurrency's essential volatility.
How Do Market Cycles Affect Cryptocurrency Price Prediction Accuracy?
Market cycles greatly impact cryptocurrency price prediction accuracy, with different phases requiring distinct analytical approaches.
During accumulation periods, on-chain metrics like declining exchange reserves provide reliable signals, whereas bubble phases exhibit diminishing predictive reliability due to irrational exuberance.
Technical indicators like the Pi Cycle Top perform best near cycle peaks, while models incorporating halving events and institutional capital flows demonstrate improved accuracy across full market cycles, particularly in the 60-80% drawdown patterns that remain consistent across major corrections.
Can Social Sentiment Analysis Accurately Forecast Crypto Price Movements?
Social sentiment analysis demonstrates moderate effectiveness in forecasting cryptocurrency price movements, with research indicating that LSTM models incorporating sentiment data achieve RMSE as low as 434.87.
While Twitter sentiments correlate strongly with price volatility and can predict directional trends, limitations persist due to data noise, neutrality bias in sentiment tools, and extreme market volatility.
Multi-source data amalgamation, combining social media, news articles, and search trends, considerably enhances predictive accuracy compared to single-platform sentiment analysis.
What Role Do Whale Transactions Play in Predicting Market Shifts?
Whale transactions serve like leading indicators of market shifts, with blockchain explorers and alert systems enabling real-time monitoring of large-scale movements.
These significant transactions, for instance multi-million token purchases or exchange deposits, typically precede price volatility by creating liquidity imbalances and triggering retail investor responses.
On-chain metrics revealing whale accumulation phases often signal forthcoming bullish trends, while sudden exchange inflows from prominent wallets frequently precede corrections, making systematic whale behavior analysis essential for anticipatory trading strategies.
How Do Regulatory Announcements Impact Prediction Model Effectiveness?
Regulatory announcements greatly impact model effectiveness, requiring rapid recalibration to maintain predictive accuracy.
Favorable regulatory frameworks correlate with improved model performance, whereas unfavorable categories, including securities classifications or interoperability limitations, necessitate immediate adjustments to avoid prediction bias.
Hybrid models combining machine learning algorithms with regulatory trend analysis demonstrate superior adaptability during policy shifts, while the unification of high-frequency trading data and blockchain metrics enables more precise capture of market reactions to regulatory developments.